Shapes of Distributions
Prerequisites
Distributions,
Measures of Central Tendency, Variability
Learning Objectives
- Compute skew using two different formulas
- Compute kurtosis
We saw in the section on distributions in Chapter
1 that shapes of distributions can differ in skew
and/or kurtosis.
This section presents numerical indexes of these two measures
of shape.
Skew
Figure 1 shows a distribution with a very large
positive skew. Recall that distributions with positive skew have
tails that extend to the right.
Distributions with positive skew normally have
larger means than medians. The mean and median of the baseball
salaries shown in Figure 1 are $1,183,417 are and $500,000 respectively.
Thus, for this highly-skewed distribution, the mean is more
than twice as high as the median. The relationship between skew
and the relative size of the mean and median led the statistician
Pearson to propose the following simple and convenient numerical
index of skew:
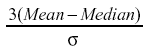
The standard deviation of the baseball salaries
is 1,390,922. Therefore, Pearson's measure of skew for this distribution
is 3(1,183,417 - 500,000)/1,390,922 = 1.47.
Just as there are several measures of central tendency,
there is more than one measure of skew. Although Pearson's measure
is a good one, the following measure is more commonly used. It
is sometimes referred to as the third moment about the mean.

Kurtosis
The following measure of kurtosis is similar to
the definition of skew. The value "3" is subtracted
to define "no kurtosis" as the kurtosis of a normal
distribution. Otherwise, a normal distribution would have a kurtosis
of 3.
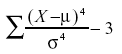
|